New Conference Paper Published
A new conference paper co-authored by Enrico Fraccaroli has been published in the proceedings of the 2025 International Conference on VLSI Design (VLSID).
We are excited to announce that our latest conference paper, titled “LO-SC: Local-Only Split Computing for Accurate Deep Learning on Edge Devices”, has been published in the proceedings of the 2025 International Conference on VLSI Design (VLSID).
Abstract
Split Computing (SC) enables deploying a Deep Neural Network (DNN) on edge devices with limited resources by splitting the workload between the edge device and a remote server. However, relying on a server can be expensive, requires a reliable network, and introduces unpredictable latency. Existing solutions for on-device DNN deployment often sacrifice accuracy for efficiency. In this paper, we study how to use the concepts from SC to split a DNN for execution on the same device without compromising accuracy. In other words, we propose Local-Only Split Computing (LO-SC), a new approach to split a DNN for execution entirely on the edge device while maintaining high accuracy and predictable latency. We formalize LO-SC as a Mixed Integer Linear Problem (MILP) and solve it using a multi-constrained ordered knapsack algorithm. The proposed method achieves promising results on both synthetic and real-world data, offering a viable alternative for accurately deploying DNNs on resource-constrained edge devices.
Details
- Title: LO-SC: Local-Only Split Computing for Accurate Deep Learning on Edge Devices
- Authors: Luigi Capogrosso, Enrico Fraccaroli, Marco Cristani, Franco Fummi, Samarjit Chakraborty
- Conference: 2025 International Conference on VLSI Design and 2024 International Conference on Embedded Systems (VLSID)
- Year: 2025
- Pages: 445-450
- Keywords: Deep learning, Accuracy, Embedded systems, Source coding, Artificial neural networks, Very large-scale integration, Prediction algorithms, Servers, Reliability, Split Computing, Knapsack Problem, Deep Neural Networks, Edge Devices
Links
- DOI: 10.1109/VLSID64188.2025.00089
- Open Access Version: Read Here
- Source Code: GitHub Repository
This paper introduces Local-Only Split Computing (LO-SC), a novel technique for deploying deep learning models on edge devices without requiring a remote server. By applying a multi-constrained knapsack optimization, the approach allows for efficient, accurate, and low-latency execution of deep neural networks while ensuring predictable performance.
We extend our gratitude to all collaborators and contributors for their efforts. For further details, please explore the links provided above.
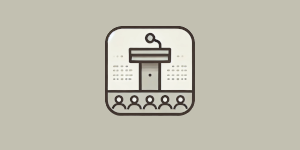
New Conference Paper Published
February 28, 2025
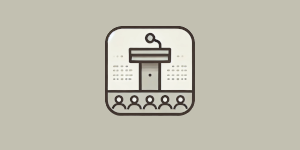
New Conference Paper Published
February 20, 2025
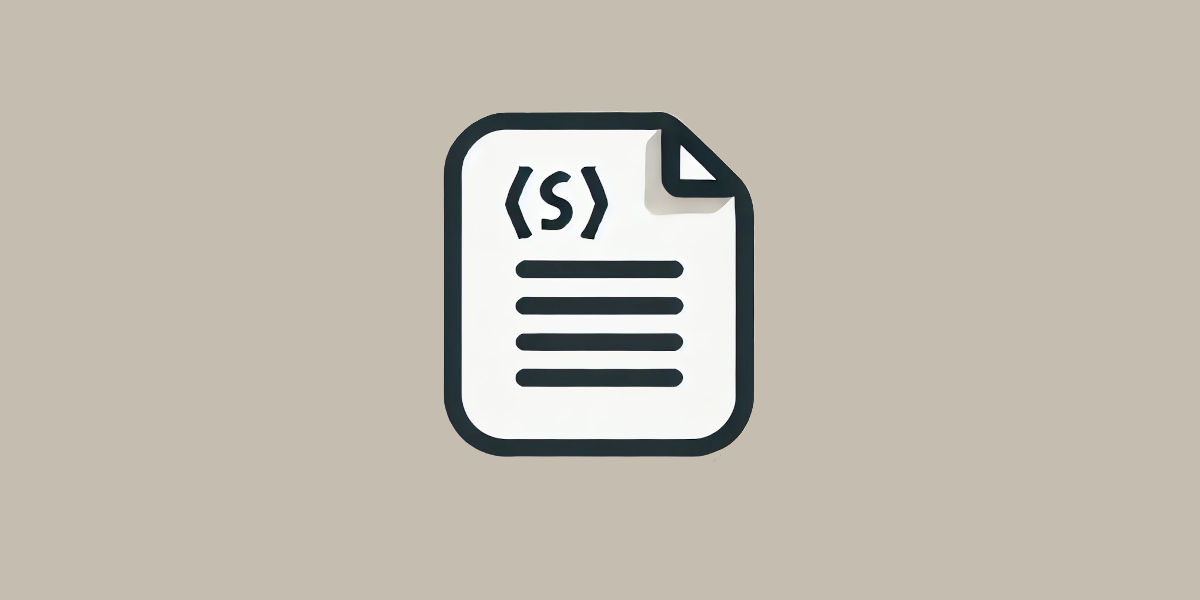
FlexMan: A New Adaptive Scheduling and Optimization Library Released
January 23, 2025
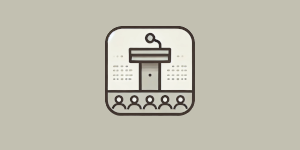
New Conference Paper Published
October 23, 2024
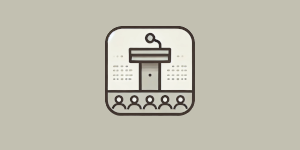
New Conference Paper Published
September 04, 2024
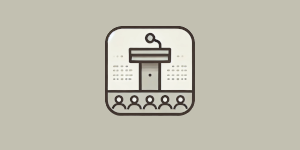
New Conference Paper Published
September 04, 2024
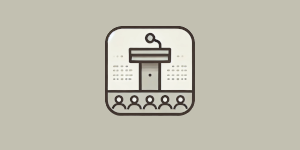
New Conference Paper Published
September 04, 2024
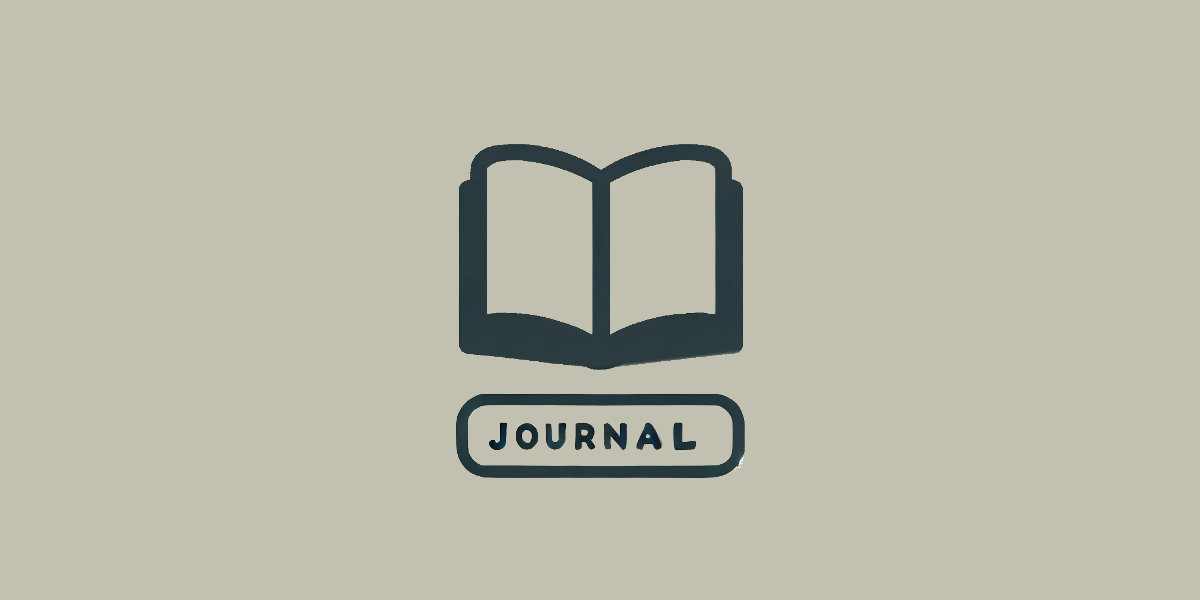
New Journal Article Published
July 25, 2024
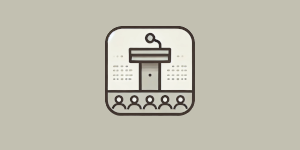
New Conference Paper Published
July 03, 2024
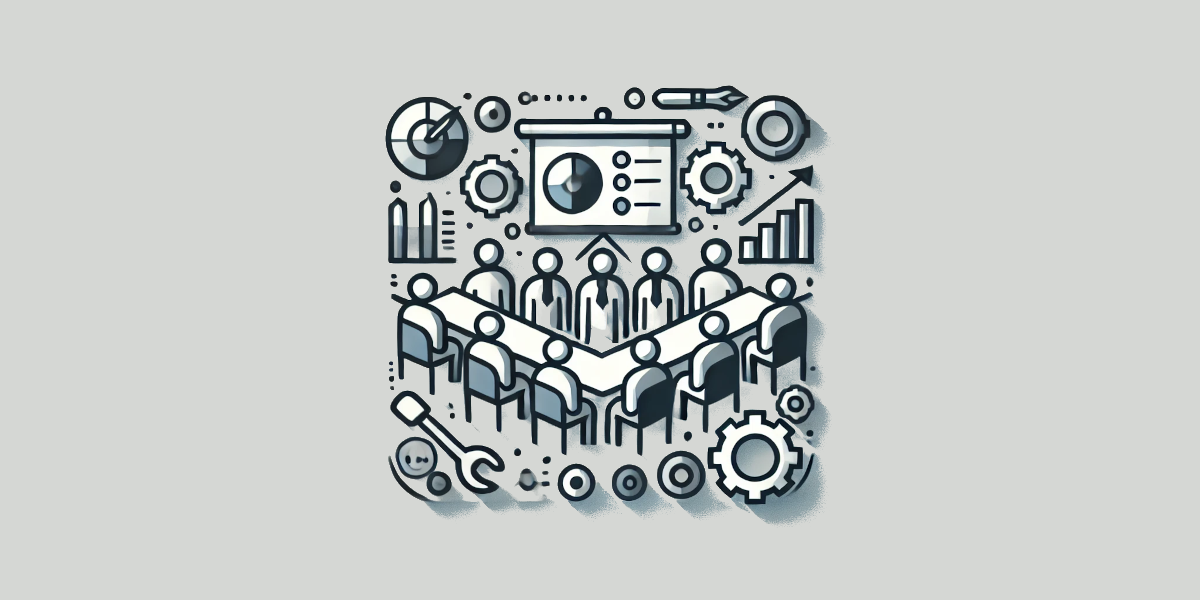
Workshop
April 15, 2024
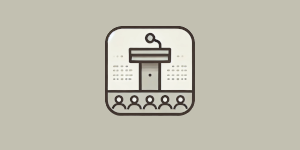
New Conference Paper Published
April 09, 2024
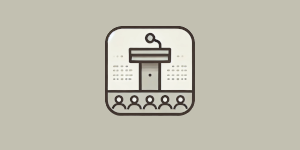
New Conference Paper Published
March 25, 2024
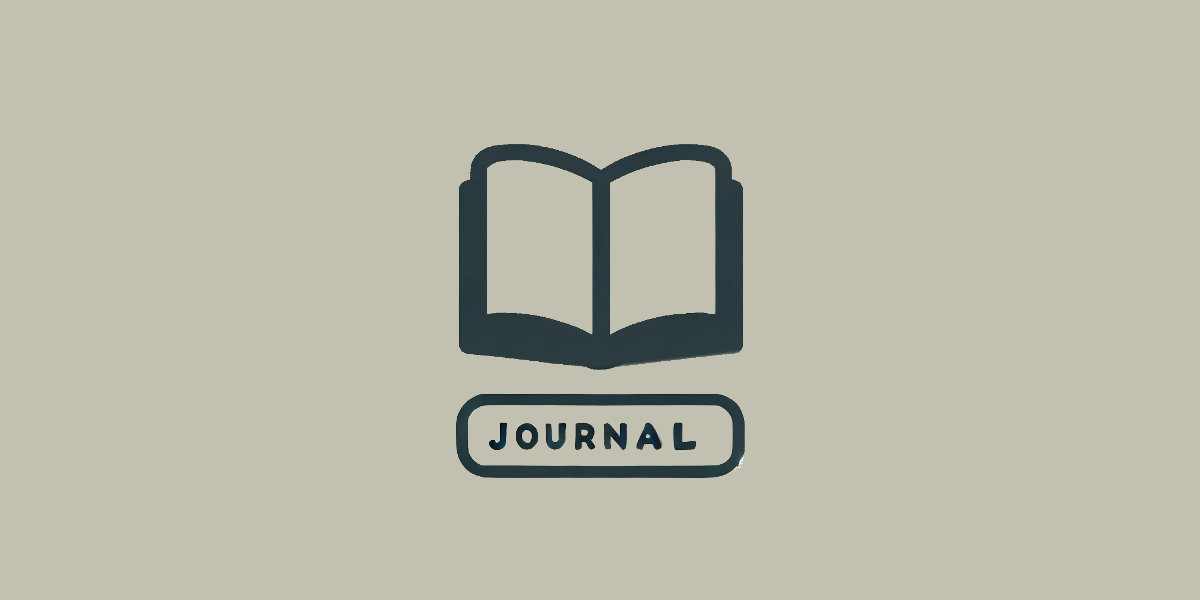
New Journal Article Published
December 21, 2023
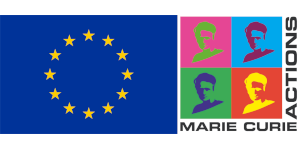